Enhancing Business Processes with Efficient Labeling Training Data
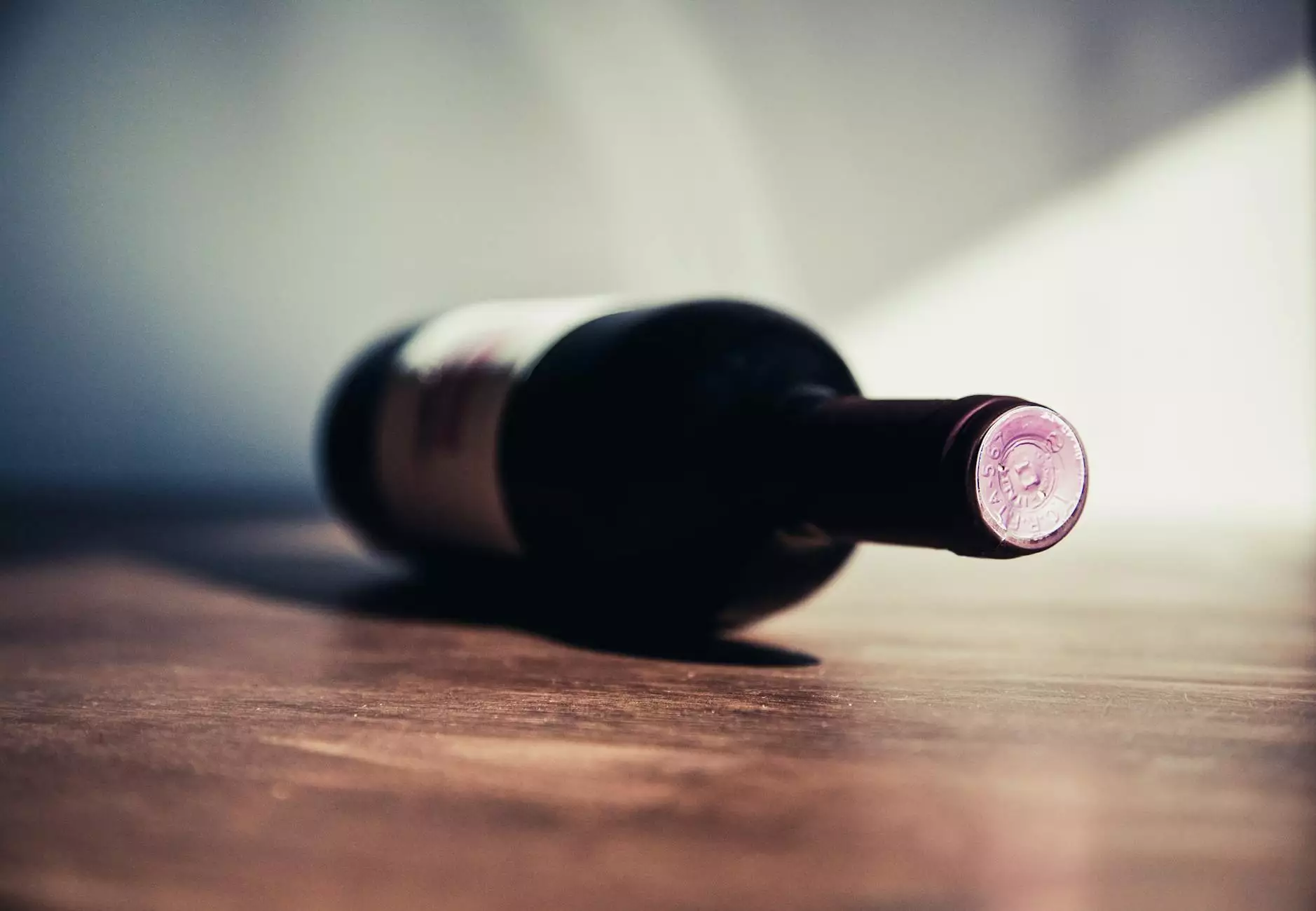
Understanding the Importance of Labeling Training Data
In the age of information, where artificial intelligence and machine learning are becoming crucial drivers for businesses, the need for accurate and comprehensive data is paramount. Labeling training data is an essential step for any organization that seeks to leverage the power of AI. This process involves annotating raw data with meaningful labels, allowing machine learning algorithms to learn and make predictions.
The Role of Data Annotation Tools
Data annotation tools play a vital role in the process of labeling training data. They provide a user-friendly interface for annotators to assign labels to various types of data including images, videos, text, and audio. The effectiveness of these tools can greatly influence the quality of the labeled data, which in turn affects the performance of AI models.
Types of Data Annotation Tools
- Image Annotation Tools: These tools help in labeling objects within images, which is especially useful in fields like autonomous driving and facial recognition.
- Text Annotation Tools: Facilitating the tagging of text data, these tools are invaluable in natural language processing applications.
- Video Annotation Tools: Used for labeling specific parts of video clips, which is beneficial for action recognition and behavior analysis.
- Audio Annotation Tools: These tools allow the transcription and labeling of audio files for applications like speech recognition.
Choosing the Right Data Annotation Platform
When it comes to labeling training data, selecting the right platform is crucial for optimizing the annotation process. An effective data annotation platform should offer the following features:
Key Features of an Effective Data Annotation Platform
- User-Friendly Interface: A platform with an intuitive interface is essential for efficient data labeling.
- Collaboration Tools: Features that allow multiple users to collaborate on labeling tasks can significantly reduce time and increase productivity.
- Quality Control Mechanisms: Implementing checks to ensure the accuracy of annotations is vital for high-quality datasets.
- Integration Capabilities: The ability to integrate with existing systems enhances workflow and productivity.
- Scalability: A platform that scales as your data needs grow is essential for long-term success.
The Impact of High-Quality Labeling Training Data on Business Success
The quality of the labeling training data directly correlates with the success of AI and machine learning projects. High-quality labeled data leads to better model accuracy, which in turn allows businesses to make informed decisions and enhance operational efficiency. Companies that invest in robust data annotation processes are more likely to outperform their competitors who overlook this critical requirement.
Real-World Applications of Labeling Training Data
Various industries are reaping the benefits of effective labeling training data. Here are some notable sectors:
Healthcare
In the healthcare sector, labeled data is used to train models that can predict patient outcomes, identify diseases from medical imaging, and streamline hospital operations. Accurate labeling training data ensures that AI models can make reliable predictions, ultimately leading to improved patient care.
Automotive
With the rise of autonomous vehicles, the automotive industry relies heavily on labeling training data for developing perception algorithms that recognize objects, pedestrians, and road signs. This not only enhances vehicle safety but also accelerates the development of self-driving technologies.
Retail
The retail industry uses labeling training data to enhance customer experiences through personalized recommendations, inventory management, and demand forecasting. By leveraging accurately labeled data, retailers can tailor their offerings to meet customer preferences and enhance sales.
Strategies for Effective Labeling Training Data
To ensure high-quality labeled data, businesses can employ several strategies:
Investing in Training and Resources
It is crucial to invest in training annotators and providing them with the resources they need to perform their tasks effectively. Skilled annotators understand the nuances of data and can ensure accurate labeling.
Utilizing Pre-trained Models
Leveraging pre-trained models can aid in the annotation process, as these models may already have a fundamental understanding of the data context, reducing the workload of annotators.
Implementing Feedback Loops
Establishing a feedback loop where annotators can improve their labeling processes based on model performance and user feedback will lead to continuous improvement in the quality of labeling training data.
Challenges in Labeling Training Data
Despite the many advantages, businesses face several challenges in the labeling of training data:
Volume and Complexity of Data
The sheer volume of data that needs to be labeled can be overwhelming. Additionally, as data grows more complex, ensuring consistent and accurate labels becomes increasingly difficult.
Subjectivity in Annotation
Certain types of data, especially in fields like sentiment analysis and image classification, involve subjective interpretation, which can lead to inconsistencies in labeling.
Resource Allocation
Balancing the resources required for accurate labeling with other operational needs can strain a business's capacity. Companies must strategically allocate personnel and technology to tackle this challenge.
Conclusion: The Future of Labeling Training Data in Business
As businesses continue to harness AI and machine learning, the significance of quality labeling training data cannot be overstated. By adopting efficient data annotation tools and platforms, organizations can streamline their workflows, overcome challenges, and drive meaningful insights from their data. The future of business innovation lies in the accuracy and effectiveness of their data, making investment in labeling training data an indispensable strategy for any forward-thinking company.