The Significance of Agriculture Dataset for Machine Learning
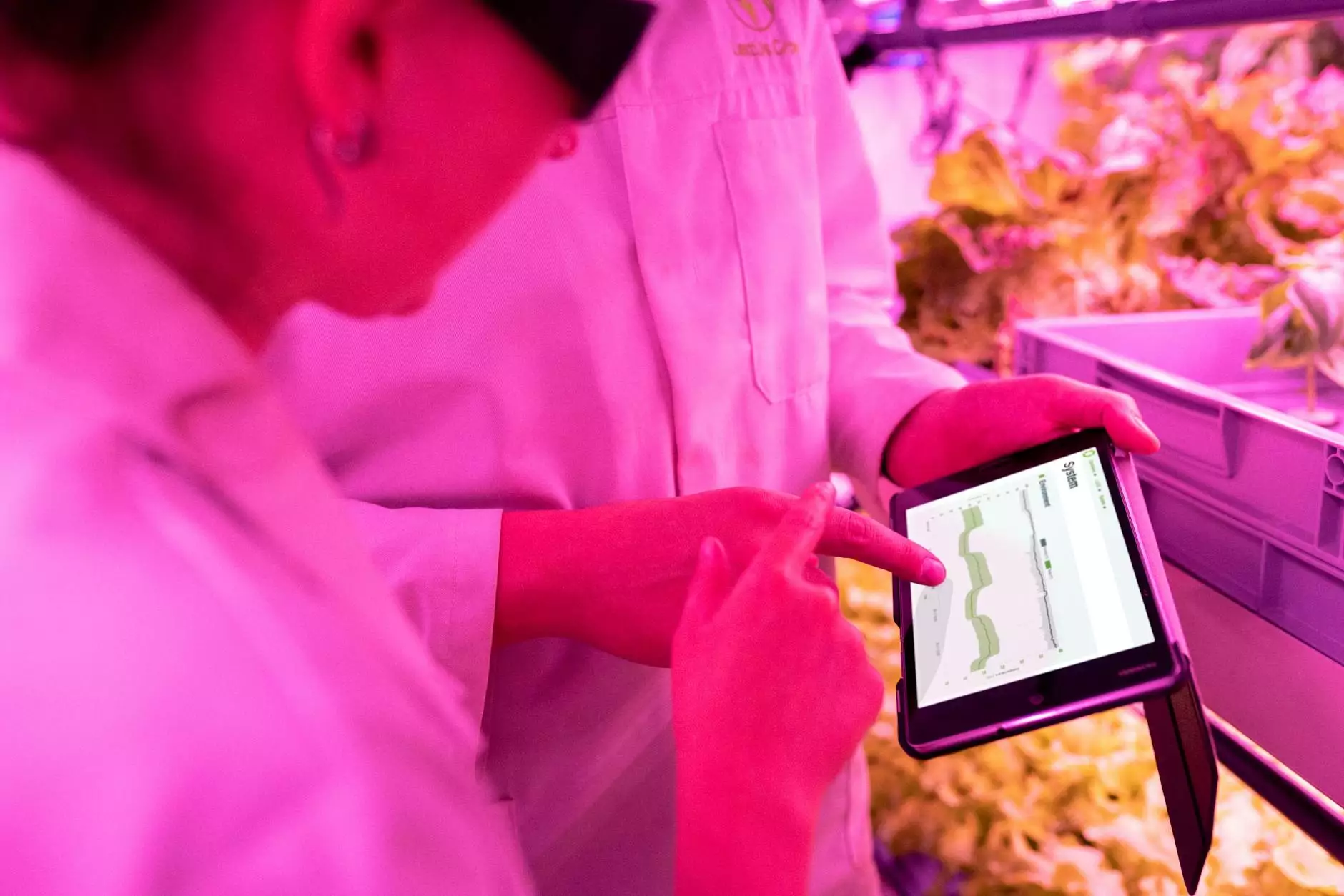
As the world continues to advance in technology, various industries have embraced the power of data-driven insights to enhance their operations. In the realm of agriculture, the utilization of datasets for machine learning has revolutionized traditional farming practices. This article delves into the importance of agriculture dataset for machine learning and how it is shaping the future of the farming industry.
Understanding Agriculture Datasets
First and foremost, let's define what agriculture datasets are. These datasets consist of a vast array of information pertaining to various aspects of agriculture, ranging from crop yields and weather patterns to soil composition and pest control measures. By leveraging this data, farmers and agricultural experts can gain valuable insights that aid in making informed decisions to optimize productivity and sustainability.
The Role of Machine Learning
Machine learning algorithms play a crucial role in analyzing agriculture datasets. By applying sophisticated computational models, these algorithms can identify patterns, trends, and correlations within the data that may not be apparent to the human eye. This enables farmers to predict outcomes, mitigate risks, and enhance efficiency in their farming practices.
Benefits of Using Agriculture Datasets for Machine Learning
- Enhanced Decision-Making: Agriculture datasets empower farmers to make data-driven decisions based on real-time information and predictive analytics.
- Optimized Resource Utilization: By analyzing datasets, farmers can optimize the use of resources such as water, fertilizers, and pesticides, leading to improved yield and reduced wastage.
- Sustainable Farming Practices: Machine learning models can help in implementing sustainable farming practices by minimizing environmental impact and promoting biodiversity.
- Early Disease Detection: Datasets can aid in early detection of crop diseases and pest infestations, allowing farmers to take timely preventive measures.
Challenges and Opportunities
While the integration of agriculture datasets and machine learning offers numerous benefits, there are also challenges that need to be addressed. These include data security concerns, algorithm accuracy, and accessibility to technology in rural farming communities. However, with the right strategies and investments in technology, these challenges can be overcome, paving the way for greater innovation in the agricultural sector.
The Future of Farming
Looking ahead, the synergy between agriculture datasets and machine learning holds immense potential for the future of farming. By harnessing the power of data analytics, farmers can drive productivity, sustainability, and profitability in the agricultural industry. This transformative approach not only benefits individual farmers but also contributes to global food security and environmental preservation.
Embrace the era of data-driven agriculture and unlock new possibilities for growth and innovation in the farming sector. Agriculture dataset for machine learning is not just a trend; it is a powerful tool that can shape the future of farming for generations to come.